Success in outbound sales depends on understanding customer conversations. Sales teams are constantly seeking ways to improve their performance, and one of the most effective methods is through the analysis of sales calls. Our Sales Call Analysis leverages the power of Gemini AI to analyze B2B sales calls, providing actionable insights that can transform how sales teams operate. In this blog post, we’ll explore what we analyzed, the methodologies employed, and the results we achieved.
What We Analyzed
Our analysis focused on several key aspects of B2B sales calls, each designed to uncover insights that can enhance sales strategies:
Call Metrics: We examined key performance indicators (KPIs) such as call duration, talk ratios, and engagement scores. These metrics help assess the effectiveness of sales conversations and identify areas for improvement.
Conversation Flow: Understanding the structure of conversations is vital. We analyzed the types of questions asked, how objections were handled, and key moments that influenced the outcome of the calls. This analysis helps identify successful patterns and areas where sales representatives may struggle.
Success Indicators: We evaluated factors that contribute to successful calls, such as qualification scores, clarity of next steps, and conversion probabilities. By understanding what leads to success, we can better train sales teams to replicate these behaviors.
Feature Analysis: Our product offers various features designed to enhance the sales process. We specifically looked at how these features were discussed during calls, measuring customer interest and feedback. This analysis helps prioritize product development and marketing efforts.
Sentiment Analysis: Tracking the sentiment of both the sales representative and the customer throughout the conversation provides insights into emotional engagement. Understanding how customers feel during calls can inform strategies for improving customer relationships.
Methodology of B2B Sales Calls with AI
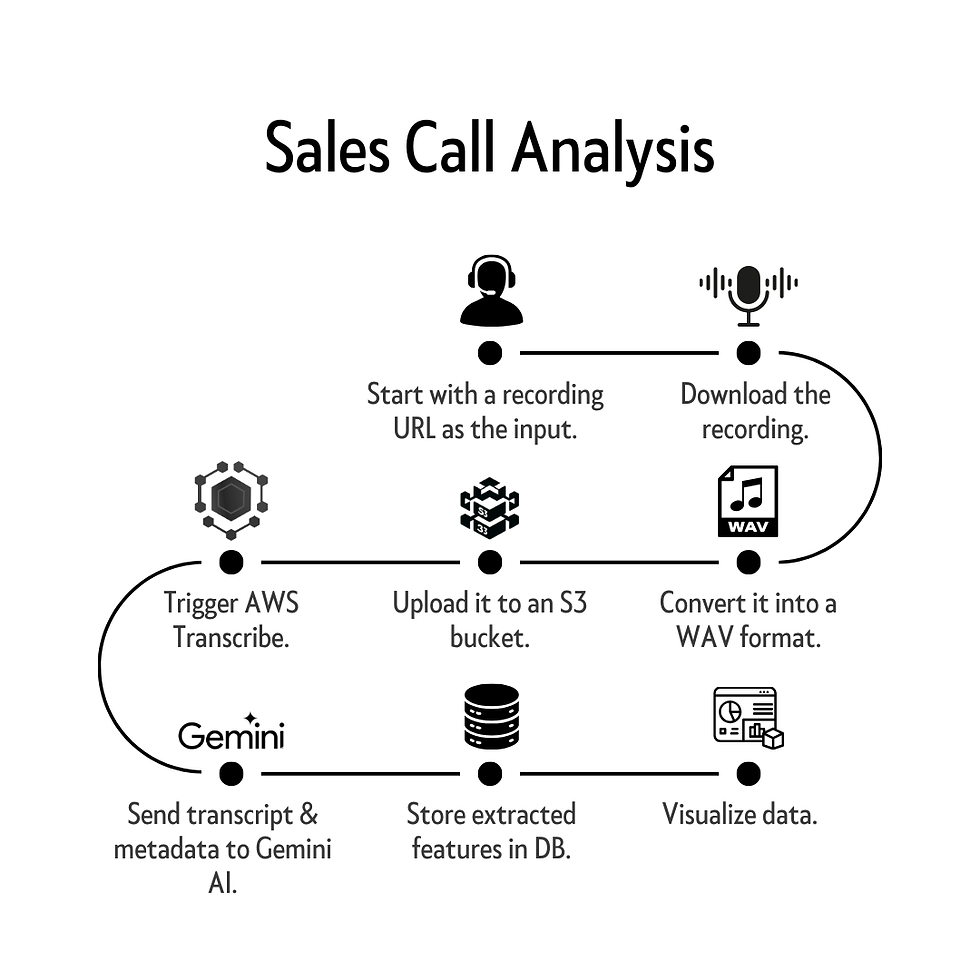
To conduct this comprehensive analysis, we developed a robust system that integrates various technologies and methodologies:
Data Collection
The Python script leverages AWS Boto3 library to automate call recording analysis. It extracts recording URLs, downloads audio files, and converts them to WAV format. After uploading processed files to an S3 bucket, the script triggers AWS Transcribe for transcription, storing the JSON output in S3 and the database. This workflow enables efficient, automated processing of sales call recordings.
Gemini AI Integration
The transcription data was retrieved from the database, complete with associated metadata like participant details and timestamp information. This comprehensive dataset was then processed through the large language model (LLM), specifically Gemini 1.5 Pro, enabling a rich, contextual analysis that went beyond just the raw transcript text. By combining transcription data with metadata, the LLM could generate more precise and nuanced insights about the sales conversations.
Database Management
A data pipeline was configured to capture and store the LLM powered analysis results directly into the database. The pipeline ensures that the AI-generated insights are systematically logged, with each entry linked to its corresponding sales call recording and transcription. This structured approach allows for easy retrieval, tracking, and further analysis of the insights generated by the Gemini AI model.
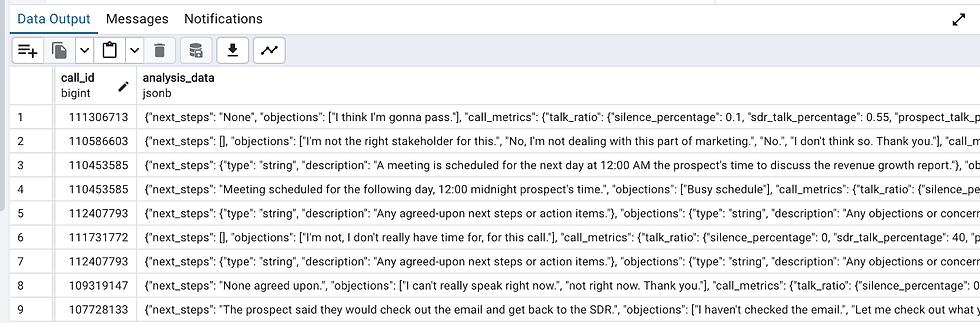
Feature Analysis
A significant part of our analysis involved examining how specific product features were discussed during sales calls. We created a structured prompt for Gemini 1.5 Pro to ensure that it focused on key features such as data analysis, intelligent segmentation, personalized journeys, reports & analytics, and integrations. By analyzing customer reactions and interest levels, we could identify which features resonated most with prospects.
Results
The analysis yielded several key findings that can help sales teams enhance their performance:
Identifying Key Success Factors
One of our analysis’s most significant outcomes was identifying key success factors in sales calls. We discovered that calls with a clear structure and defined next steps had a significantly higher conversion rate. Sales representatives who effectively engaged customers with relevant questions were more likely to schedule meetings. This insight emphasizes the importance of training sales teams on effective communication techniques and structured call flows.
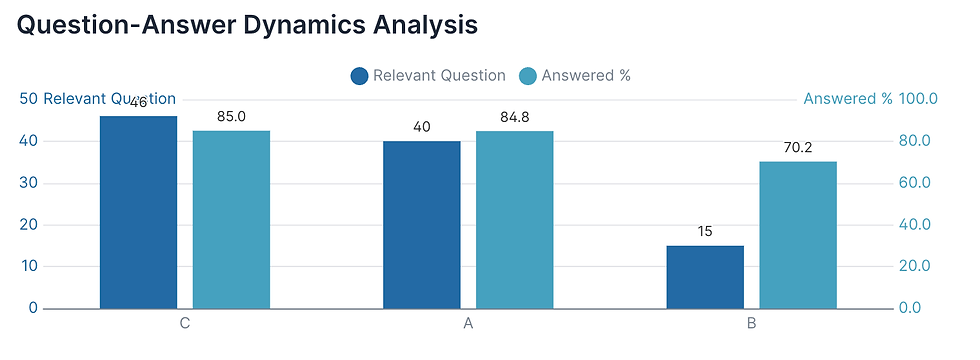
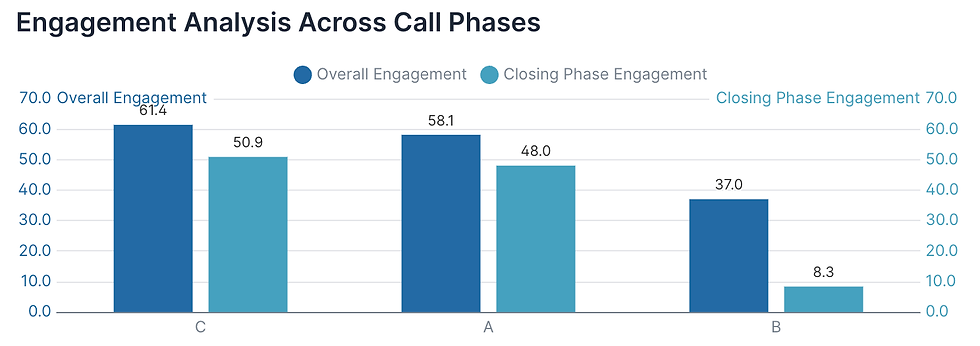
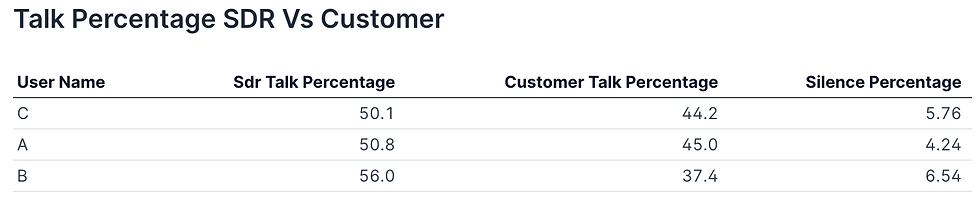
Feature Effectiveness
The analysis revealed which product features garnered the most interest from customers. For instance, features related to data analysis and personalized journeys received the highest engagement scores. This information is invaluable for product development and marketing strategies, as it allows us to focus on enhancing features that customers find most beneficial.
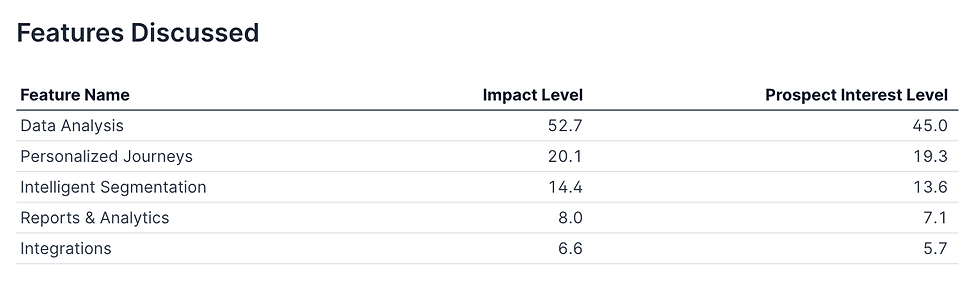
Sentiment Insights
By tracking sentiment throughout the calls, we identified moments of high engagement and resistance. For example, we found that when sales representatives used positive language and actively listened to customer concerns, the overall sentiment of the call improved. This insight can inform training programs aimed at enhancing emotional intelligence and rapport-building skills among sales teams.
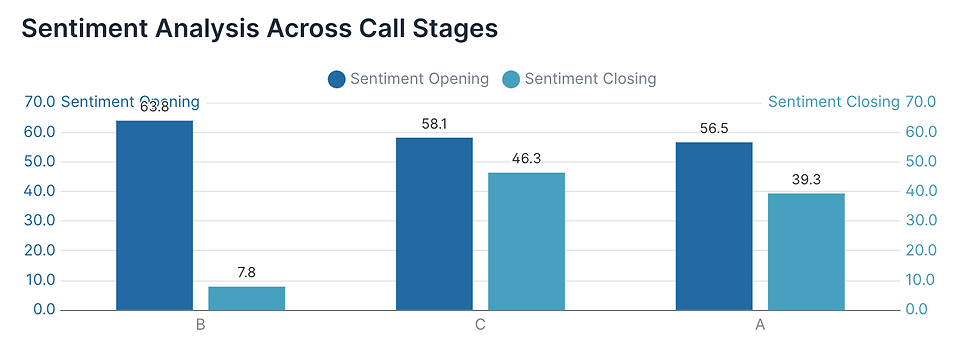
Postive Factors for SDR

Actionable Recommendations
Based on the analysis, we provided tailored recommendations for improving sales strategies. These included:
Training on Objection Handling: Equip sales representatives with techniques to effectively address common objections and turn them into opportunities.
Enhancing Product Knowledge: Ensure that sales teams are well-versed in product features and benefits, enabling them to communicate value effectively.
Refining Communication Techniques: Encourage active listening and empathetic responses to foster stronger connections with prospects.
Improvement Suggestions For SDR

Contact Fit Recommendation Report
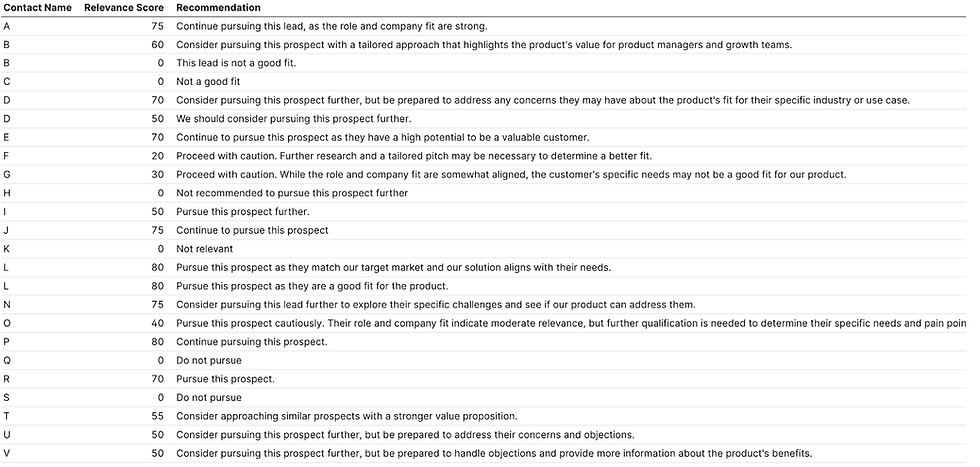
Conclusion
Our AI-powered analysis of B2B sales calls has opened new avenues for understanding customer interactions. By leveraging the capabilities of large language models (LLMs) along with AWS Transcribe for accurate data management and feature analysis, we can provide sales teams with targeted insights to enhance their performance. These advanced tools allow for a deeper understanding of customer interactions and sales dynamics, facilitating more informed decisions and driving improved sales results.The findings from our analysis not only highlight the importance of structured conversations and effective objection handling but also emphasize the value of understanding customer sentiment.
As we continue to enhance our services, we look forward to sharing more insights and success stories from our users. The future of sales analytics is bright, and we are excited to be at the forefront of this transformation. Stay tuned for updates as we explore new ways to empower sales teams and enhance customer relationships!
Comments